Tech
AI and Machine Learning for Photonic Systems: 7 Ways They Are Transforming the Future of Optics
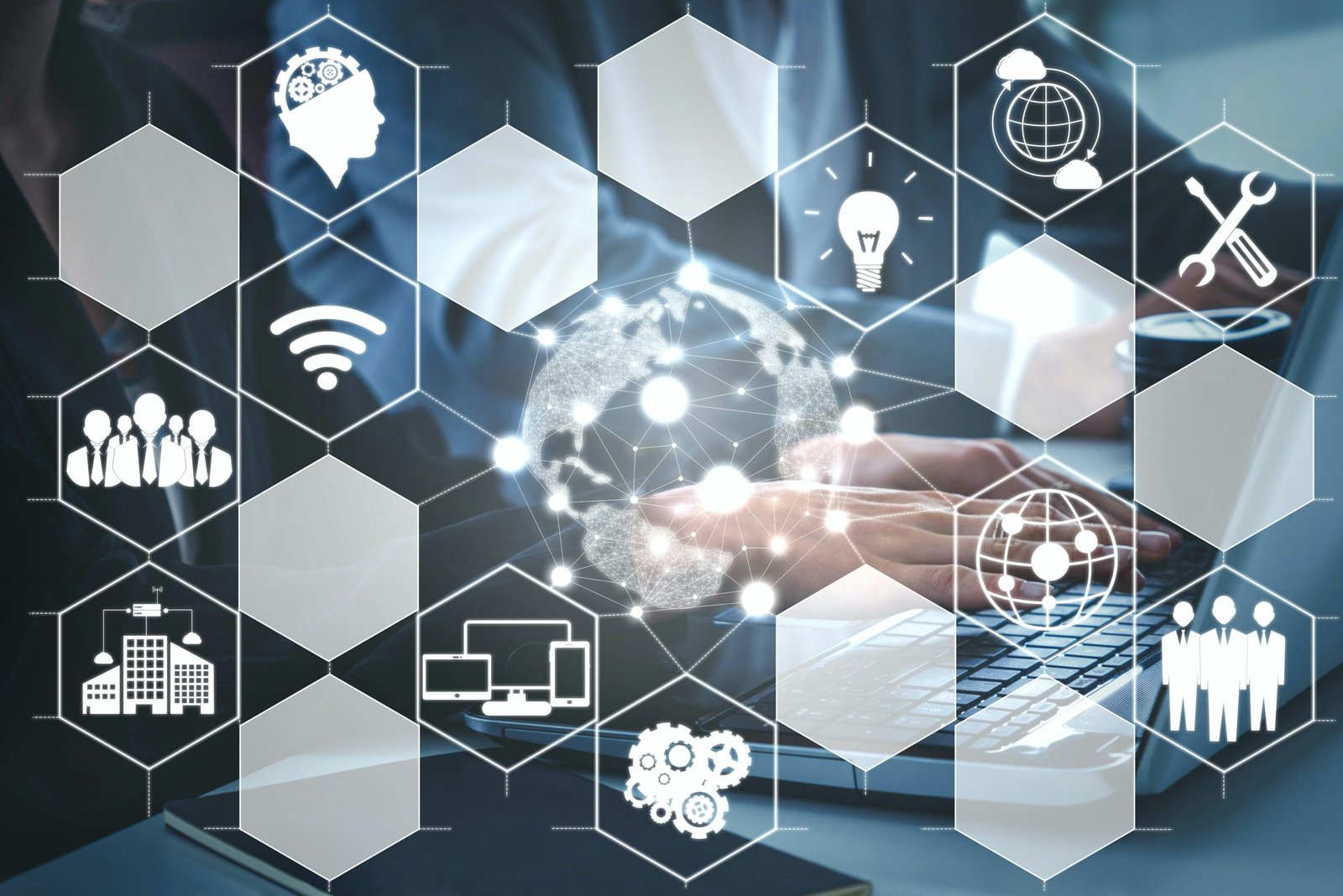
Table of Contents
Explore how AI and machine learning for photonic systems are revolutionizing photonics. Discover the transformative impact these technologies have on optical communication, sensor technology, and quantum systems in this detailed guide.
AI and Machine Learning for Photonic Systems: A New Era of Optics and Innovation
In the realm of photonics, the integration of AI and machine learning for photonic systems has brought about transformative changes. Photonic systems, which utilize light to process information, have traditionally been limited by hardware constraints and complex design challenges. However, the synergy between AI, machine learning (ML), and photonics has opened up new possibilities for innovation, efficiency, and performance. In this post, we will dive deep into how AI and ML are shaping the future of photonic systems and their various applications, from communications to sensing technologies.
Understanding Photonic Systems: The Basics
Before we explore the powerful impact of AI and ML, let’s first understand what photonic systems are. Simply put, photonics refers to the science of generating, controlling, and detecting light, typically in the form of photons. Photonic systems are used in a wide range of applications, from fiber-optic communications and medical imaging to quantum computing and sensing technologies. These systems rely on optical components like lasers, lenses, and detectors to transmit and process data at incredibly high speeds and precision.
As the demand for faster, more efficient data transmission and processing grows, traditional photonic systems are facing challenges in meeting these needs. This is where AI and machine learning come into play, helping to optimize, design, and even predict the behavior of photonic devices.
How AI and Machine Learning Are Revolutionizing Photonics
The integration of AI and machine learning for photonic systems is transforming the way these systems are designed, operated, and optimized. One of the main benefits of machine learning is its ability to process and analyze massive amounts of data in real-time. In photonic systems, this capability can be used to improve everything from signal processing to optical device design.
AI in Photonic System Design and Optimization
Traditionally, designing and optimizing photonic systems required extensive trial and error, as well as sophisticated simulations. However, AI-driven algorithms can now automate much of this process, significantly reducing time and cost. Machine learning models can predict the performance of photonic devices, identify optimal configurations, and even suggest improvements based on the analysis of existing data.
For example, AI algorithms can analyze light propagation through optical fibers or waveguides, optimizing signal integrity and minimizing loss during transmission. In semiconductor manufacturing, machine learning can help to optimize the design of photonic integrated circuits (PICs), leading to more compact and efficient devices.
By combining the power of AI with photonic systems, engineers can not only design systems that meet specific performance requirements but also predict and correct any issues before they arise. This helps improve system reliability, reduce downtime, and accelerate innovation in the field.
Applications of AI and Machine Learning in Photonics
AI and machine learning are making their presence felt across various photonic applications, with significant improvements in optical communication, sensor technology, and even quantum computing.
Enhancing Optical Communication Systems with AI
One of the most prominent applications of AI and machine learning for photonic systems is in optical communication. Optical communication, which uses light to transmit data over long distances, has seen exponential growth thanks to the demand for faster internet speeds and improved connectivity. AI plays a pivotal role in optimizing these communication systems.
Machine learning algorithms can be used to improve signal processing in optical networks, reducing noise and interference. In fiber-optic communication, for example, AI can dynamically adjust transmission parameters to optimize data rates and minimize signal degradation. This is particularly useful in dense networks where multiple signals are being transmitted simultaneously, requiring precise control to avoid overlap and interference.
Moreover, AI is helping to enable the next generation of optical communication systems, such as quantum communication, which relies on the unique properties of quantum states of light to provide ultra-secure data transmission. Machine learning models are being used to design quantum key distribution systems, enhancing the efficiency and security of these networks.
AI-Powered Sensing Technologies
Sensing is another area where AI and machine learning for photonic systems have made significant strides. Photonic sensors are used in a wide range of industries, from healthcare to environmental monitoring, providing highly accurate measurements based on light interactions. By integrating AI into these systems, we can achieve more precise, real-time data analysis.
For example, in medical diagnostics, AI-powered photonic sensors are being used for early detection of diseases through non-invasive imaging techniques. These sensors can capture minute changes in the light reflected or scattered by tissues, and machine learning algorithms can analyze the data to detect abnormalities such as tumors or disease markers.
In environmental monitoring, AI-driven photonic sensors are improving the detection of pollutants in the air and water, enabling more accurate and timely responses to environmental issues.
The Role of AI and Machine Learning in Quantum Photonics
Quantum photonics is one of the most exciting and rapidly developing fields in photonics today. By leveraging the unique properties of quantum mechanics, photonic systems can be used for applications such as quantum computing, quantum cryptography, and quantum sensing. Here, AI and machine learning are playing a crucial role in optimizing quantum photonic devices and systems.
Optimizing Quantum Photonic Devices with AI
Quantum photonic devices, such as single-photon sources and quantum detectors, are notoriously difficult to design and manufacture due to the complex nature of quantum behavior. AI and machine learning models are being used to simulate quantum processes, helping researchers better understand how quantum systems behave and predict the most efficient configurations for quantum devices.
Machine learning is also helping in quantum error correction, a critical challenge in quantum computing. By using AI to analyze and correct errors in quantum computations, researchers are moving closer to developing stable and scalable quantum computers.
Machine Learning for Quantum Cryptography
Quantum cryptography offers a level of security that classical encryption methods cannot achieve, making it a promising field for securing communications. AI is helping optimize quantum cryptography protocols, such as quantum key distribution, by analyzing vast amounts of quantum data and improving error rates.
Challenges and Future Outlook
While the integration of AI and machine learning into photonic systems holds immense promise, there are still challenges to overcome. One of the biggest hurdles is the need for large amounts of high-quality data to train machine learning models. In many cases, obtaining this data can be difficult due to the complexity of photonic systems and the specialized nature of the field.
Another challenge is the integration of AI with existing photonic infrastructure. Many photonic systems are still built using traditional design methods, and incorporating AI-driven optimization requires significant changes to the design process.
However, as both AI and photonics continue to evolve, the potential for innovation is boundless. Researchers are exploring new ways to enhance the integration of AI and photonics, such as through the use of neuromorphic computing and deep learning models. As these technologies continue to mature, the future of AI and machine learning for photonic systems looks incredibly promising.
Conclusion
The integration of AI and machine learning for photonic systems is transforming the field of photonics, opening up new opportunities for faster, more efficient, and more innovative technologies. From optical communication to quantum photonics, AI is enhancing the performance and capabilities of photonic systems, making them smarter, more precise, and more adaptable.
As research in both AI and photonics continues to advance, we can expect even more groundbreaking developments in the years to come. For industries ranging from telecommunications and healthcare to quantum computing, the convergence of AI and photonics is set to redefine what’s possible in the world of technology.
read more:Best Gaming Headphones: Top 10 Picks for 2024 | Ultimate Sound Experience

-
Blog10 months ago
Jessica Dorrell: The Scandal, Life Beyond the Headlines, and Career Path
-
Blog9 months ago
Alia Bhatt’s Daughter: A New Chapter in the Bollywood Star’s Life
-
Blog10 months ago
Rick Hoffman’s Private Life and Wife: Insights into the Suits Actor’s Personal Journey
-
Lifestyle8 months ago
A Guide to Exclusive Lifestyle Events
-
Blog8 months ago
M4U Free Movies: Your Guide to Free Online Streaming
-
Business8 months ago
Cherry Financing: 7 Powerful Strategies to Secure the Best Car Loan Today
-
Blog9 months ago
Angel Reese’s Net Worth: Rising Star in Women’s Basketball
-
Blog9 months ago
M4uMovies: Exploring the Popular Online Streaming Platform